CrisperWhisper: A Breakthrough in Speech Recognition Technology with Enhanced Timestamp Precision, Noise Robustness, and Accurate Disfluency Detection for Clinical Applications
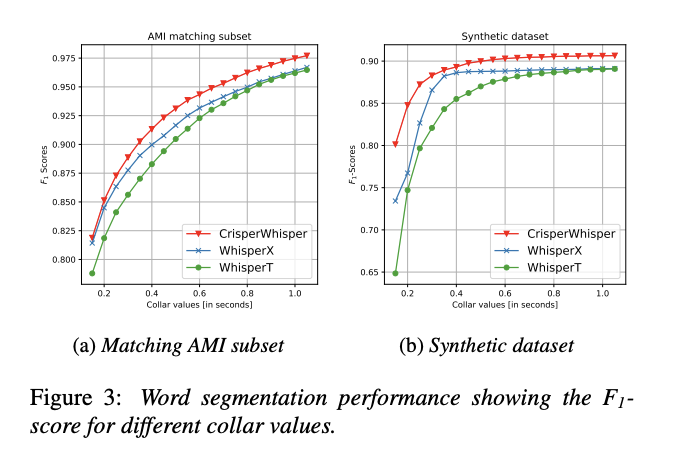
Accurately transcribing spoken language into written text is becoming increasingly essential in speech recognition. This technology is crucial for accessibility services, language processing, and clinical assessments. However, the challenge lies in capturing the words and the intricate details of human speech, including pauses, filler words, and other disfluencies. These nuances provide valuable insights into cognitive processes and are particularly important in clinical settings where accurate speech analysis can aid in diagnosing and monitoring speech-related disorders. As the demand for more precise transcription grows, so does the need for innovative methods to address these challenges effectively.
One of the most significant challenges in this domain is the precision of word-level timestamps. This is especially important in scenarios with multiple speakers or background noise, where traditional methods often need to improve. Accurate transcription of disfluencies, such as filled pauses, word repetitions, and corrections, is difficult yet crucial. These elements are not mere speech artifacts; they reflect underlying cognitive processes and are key indicators in assessing conditions like aphasia. Existing transcription models often need help with these nuances, leading to errors in both transcription and timing. These inaccuracies limit their effectiveness, particularly in clinical and other high-stakes environments where precision is paramount.
Current methods, like the Whisper and WhisperX models, attempt to tackle these challenges using advanced techniques such as forced alignment and dynamic time warping (DTW). WhisperX, for instance, employs a VAD-based cut-and-merge approach that enhances both speed and accuracy by segmenting audio before transcription. While this method offers some improvements, it still faces significant challenges in noisy environments and with complex speech patterns. The reliance on multiple models, like WhisperX’s use of Wav2Vec2.0 for phoneme alignment, adds complexity and can lead to further degradation of timestamp precision in less-than-ideal conditions. Despite these advancements, there remains a clear need for more robust solutions.
Researchers at Nyra Health introduced a new model, CrisperWhisper. This model refined the Whisper architecture, improving noise robustness and single-speaker focus. The researchers significantly enhanced word-level timestamps’ accuracy by carefully adjusting the tokenizer and fine-tuning the model. CrisperWhisper employs a dynamic time-warping algorithm that aligns speech segments with greater precision, even in background noise. This adjustment improves the model’s performance in noisy environments and reduces errors in transcribing disfluencies, making it particularly useful for clinical applications.
CrisperWhisper’s improvements are largely due to several key innovations. The model strips unnecessary tokens and optimizes the vocabulary to detect better pauses and filler words, such as ‘uh’ and ‘um.’ It introduces heuristics that cap pause durations at 160 ms, distinguishing between meaningful speech pauses and insignificant artifacts. CrisperWhisper employs a cost matrix constructed from normalized cross-attention vectors to ensure that each word’s timestamp is as accurate as possible. This method allows the model to produce transcriptions that are not only more precise but also more reliable in noisy conditions. The result is a model that can accurately capture the timing of speech, which is crucial for applications that require detailed speech analysis.
The performance of CrisperWhisper is impressive when compared to previous models. It achieves an F1 score of 0.975 on the synthetic dataset and significantly outperforms WhisperX and WhisperT in noise robustness and word segmentation accuracy. For instance, CrisperWhisper achieves an F1 score of 0.90 on the AMI disfluency subset, compared to WhisperX’s 0.85. The model also demonstrates superior noise resilience, maintaining high mIoU and F1 scores even under conditions with a signal-to-noise ratio of 1:5. In tests involving verbatim transcription datasets, CrisperWhisper reduced the word error rate (WER) on the AMI Meeting Corpus from 16.82% to 9.72%, and on the TED-LIUM dataset from 11.77% to 4.01%. These results underscore the model’s capability to deliver precise and reliable transcriptions, even in challenging environments.

In conclusion, Nyra Health introduced CrisperWhisper, which addresses timestamp accuracy and noise robustness. CrisperWhisper provides a robust solution that enhances the precision of speech transcriptions. Its ability to accurately capture disfluencies and maintain high performance in noisy conditions makes it a valuable tool for various applications, particularly in clinical settings. The improvements in word error rate and overall transcription accuracy highlight CrisperWhisper’s potential to set a new standard in speech recognition technology.
Check out the Paper. All credit for this research goes to the researchers of this project. Also, don’t forget to follow us on Twitter and LinkedIn. Join our Telegram Channel. If you like our work, you will love our newsletter..
Don’t Forget to join our 50k+ ML SubReddit
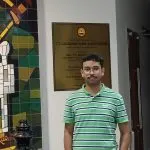
Sana Hassan, a consulting intern at Marktechpost and dual-degree student at IIT Madras, is passionate about applying technology and AI to address real-world challenges. With a keen interest in solving practical problems, he brings a fresh perspective to the intersection of AI and real-life solutions.
Comments are closed, but trackbacks and pingbacks are open.